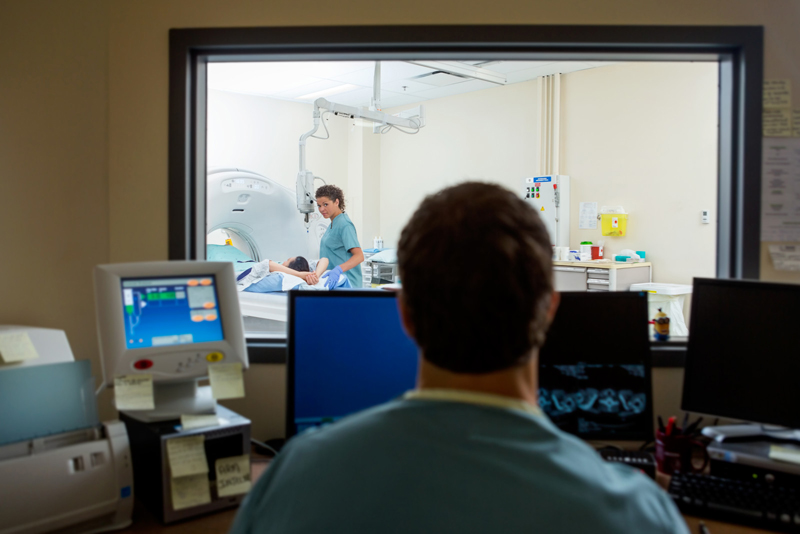
As a US based medical transcription company that provides HIPAA compliant radiology transcription services, we closely track developments in diagnostic imaging and related areas. Recent reports indicate that artificial intelligence (AI) and machine learning (ML) are creating inroads into these fields, offering radiologists many opportunities to increase workflow efficiency and productivity, improve patient care, and reduce costs. Let’s take a look at the benefits of AI applications in radiology.
Improved Diagnosis
AI promises improved diagnosis. Diagnostic tools can be trained using cutting-edge technology to read radiologic scans and tissue samples. Radiologists can take advantage of AI and ML to find abnormalities in patient scan images that could help lead physicians to arrive at the right diagnosis. In a study published in Radiology in 2018, AI was able to detect Alzheimer’s disease in brain scans six years prior to diagnosis with 98% accuracy. As advanced tools automate repetitive tasks and help radiologists prioritize cases or secondary reads according to tumor detection, they can provide patients with a more accurate diagnosis (www.itnonline.com).
Current FDA approved algorithms allow identifying suspicious pulmonary nodules, reducing CT dose, identifying intracranial haemorrhage, spinal fractures, pulmonary embolism, pneumothorax, and rib fracture. An article in Radiology Business noted how experts developed a way to use AI to overcome diagnostic challenges in pediatric elbow abnormailities by automating the elbow radiograph analysis. It’s not easy to diagnose elbow injuries in children using X-rays due to the unique features of the developing skeletal system. Also, fracture patterns in children’s elbows vary significantly depending on the child’s age and certain developmental factors. The researchers solved these problems by developing an AI elbow application model that could process multiple views of the pediatric elbow, and recognize patterns in an image and classify if something is present or not.
The BBC recently reported on a study which found that AI outperformed doctors in diagnosing breast cancer. The AI model developed by an international team was as good as the current NHS double-reading system of two doctors. An expert noted that AI promises to make breast cancer screening more accurate and efficient, reducing waiting time and worrying for patients, and providing better outcomes. However, the report points out that while AI would eliminate with the need for dual reading of mammograms by two doctors, easing pressure on their workload, it would not replace the radiologist. At least one radiologist would be in charge of diagnosis.
Continuous Learning
AI can also support continuous training of radiologists and other healthcare professionals (www.itnonline.com).AI and ML can create feedback loops – the process through which inputs of a system are plugged back. When radiologists are notified of a pathology or surgical result, this will allow radiologists to easily access previous cases with similar outcomes/diagnosis, helping them learn more and improve future processes.
Cybersecurity
Radiology practices are as vulnerable to cybersecurity threats as medical practices. The complex radiology environment comprises various systems where protected health information (PHI) is transmitted and stored. This includes RIS, PACS, imaging equipment, mobile devices, e-mails and other messaging systems, cloud storage, patient portals, and revenue cycle management systems. Moreover, many processes are managed by business associates such as medical transcription companies, medical billing and coding companies, etc. The increasing use of these systems and processes has increased risk of security breach. Radiology practices need to take steps to protect patient records and reduce the likelihood of a breach. The Imaging Technology News article recommends that radiologists should implement an effective AI security solution that includes protecting all AI-powered systems, leveraging AI to improve cybersecurity, and using AI to predict attacks.
Reimbursement
With declining reimbursement rates for image reviews, radiologists are under increasing pressure to read and interpret more images to meet their productivity goals and stay in business (www.healthanalytics.com). At the same time, the increasing amount of data that radiologists have to interpret is also leading to burnout and taking attention away from patients. The need to read images quickly can also affect accuracy of results. Misdiagnoses can be harmful and costly to patients’ health as well as to radiology business.Advanced algorithms can identify abnormal results more accurately and quickly compared to radiologists, easing their burden and overcoming reimbursement related challenges.
AI products are set to take on more complicated and complex problems in the radiology scenario. To succeed with AL and ML, radiologists need to be educated on the benefits of using AI, as well as how to use the tools correctly. Importantly, providers must also be able to identify when AI errs, says Mitchell Schnall, MD, chair of the radiology department at the Perelman School of Medicine at the University of Pennsylvania (diagnosticimaging.com).
Radiologists educate themselves on AI and practice using the tools at the the ACR AI-Lab created by the American College of Radiology’s (ACR) Data Science Institute. This tool allows radiologists to access learning videos and cases as well as to design their own to test how the technologies work.
Even with advances in technology, radiology practices will continue to need reliable transcription services to produce reports as quickly and efficiently as possible. This will allow the requesting physician to read and act upon radiology reports in a timely manner for proper patient management.